
VERtical Innovation in the Domain of Robotics Enabled by Artificial intelligence Methods
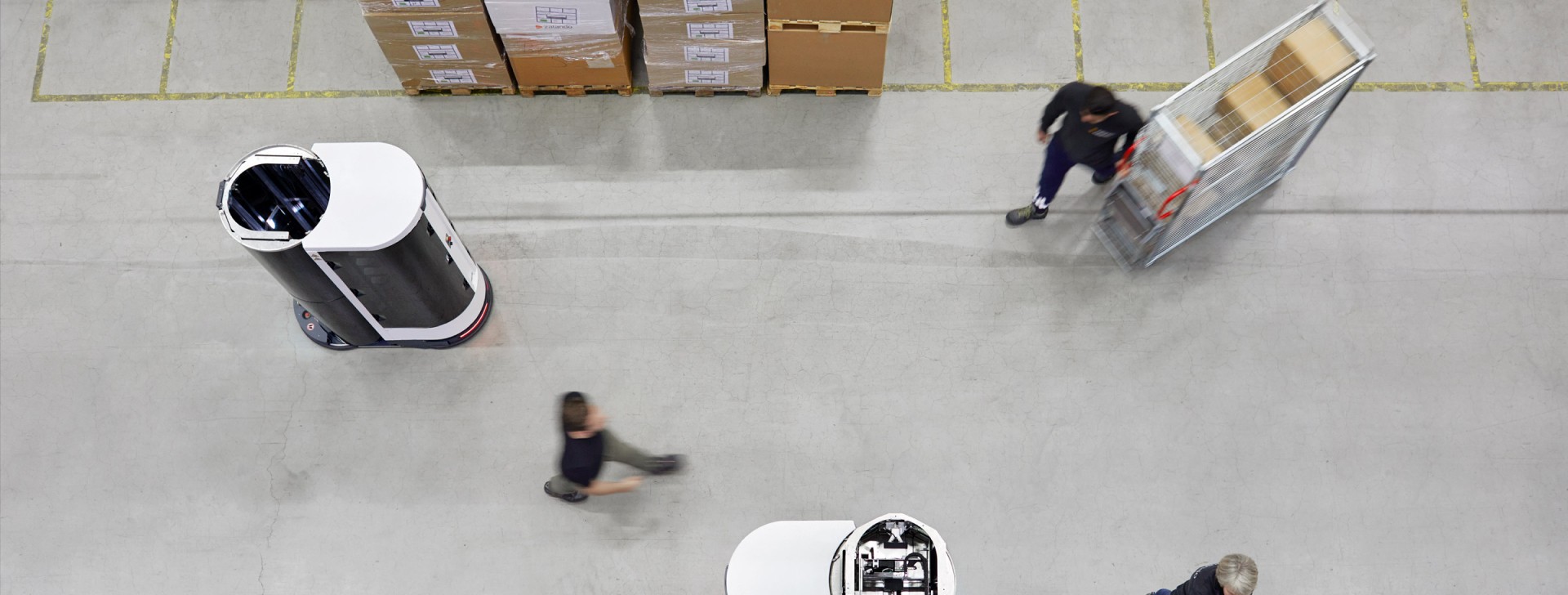
Robots act in the real world. When deploying Artificial Intelligence (AI) methods on robots, the continuous and dynamic nature of the physical world raises many challenges which are not encountered in purely digital domains such as Internet search and social networks. To tackle these challenges, the EU-funded project VeriDream builds on the DREAM and RobDream research projects to pursue a two-fold innovation strategy for AI in robotics. Its deep innovation strategy will strive to achieve high technological readiness in a set of use cases at a warehouse logistics start-up. Its broad innovation strategy will promote a wider uptake of effective innovation methods in SMEs, thus enhancing the innovation potential of SMEs in AI for robotics.
Objective
Advances in artificial intelligence (AI) are changing the business models of many companies, and creating entirely new ones. But whereas the general public associates AI predominantly with autonomous and humanoid robots, the economic impact of AI on robotics has been very limited in comparison to domains which were digitised from the start, such as Internet search and social networks. This is because acting in the physical world raises many challenges related to the variability of the real world, its continuous and dynamic nature, as well as the consequences of suboptimal or erroneous behaviour.
To address these challenges, VeriDream proposes a two-fold research and innovation strategy for AI in robotics, based on the generalisation and robustification of AI methods developed by the three research participants in two previous H2020 projects, DREAM and RobDREAM. The deep innovation strategy aims at high TRL on a set of use cases from the specific domain of warehouse logistics at the start-up Magazino.
The broad innovation strategy, pursued by Synesis and GoodAI, aims at fostering a broader uptake of DREAM methods in SMEs, also beyond the project, and even beyond robotics.
VeriDream thus aim at both concrete high-TRL innovation success stories, as well as providing experience and templates for innovation from which other European SMEs may profit.
In both strategies, our methodology is based on “”closing the innovation loop””. This means that research on AI methods is driven less by performance on static benchmarks, but rather by general methodological requirements derived from the performance on multiple industrial use cases. This will require scientific advances in state representation learning, failure discovery and resolution, and continual learning. The generalisation and robustification of DREAM methods that results from this research will have a substantial impact on the innovation potential of these methods. Demonstrating this is VeriDream’s mission.
News & Events
-
09. 11. 2022
-
08. 11. 2022
JMLR article on Stable Baselines 3
Read more -
08. 10. 2022
Game Developers Session Talk: Lessons Learned from Teaching Robots to Walk
Read more